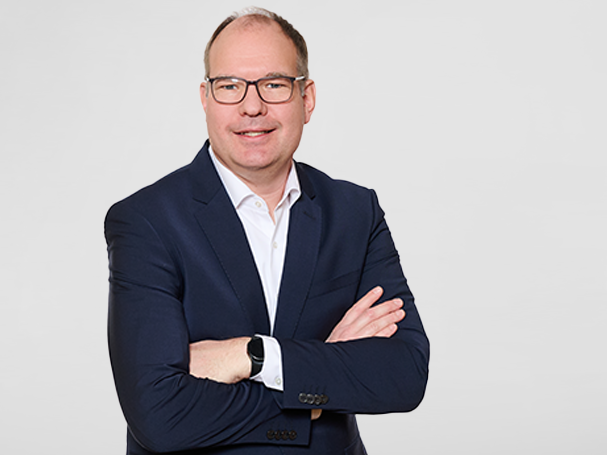
Targeted use of AI in maintenance and asset managementrealizes sustainability potential
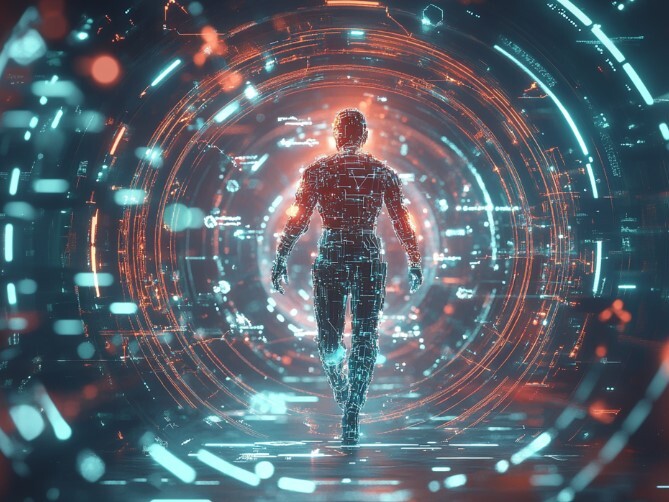
The pressure on companies to combine profitability and sustainability is increasing. In times of high energy costs and strict environmental and sustainability regulations, process efficiency and resource conservation are becoming increasingly important to survive the tough competition. Artificial intelligence offers new opportunities for improving the productivity of machines and systems, particularly in maintenance, by minimizing system downtimes and optimizing system control. Starting points are, for example, preventive maintenance with AI-supported condition monitoring and a system operation mode that is based on sustainability parameters. Compliance with these parameters is continuously monitored by AI and which also takes over the readjustment.
Asset management on the other hand uses AI in the modernization of plants and in the planning of new plants. AI supports complex repair-or-replace decisions by creating a digital twin, assists with the calculation of life cycle costs and, in particular, helps to simulate and calculate a range of different scenarios. In this way, companies can get to grips with the complex issues of profitability and sustainability and optimize their production systems from both points of view.
AI complements proven methods of value-oriented maintenance
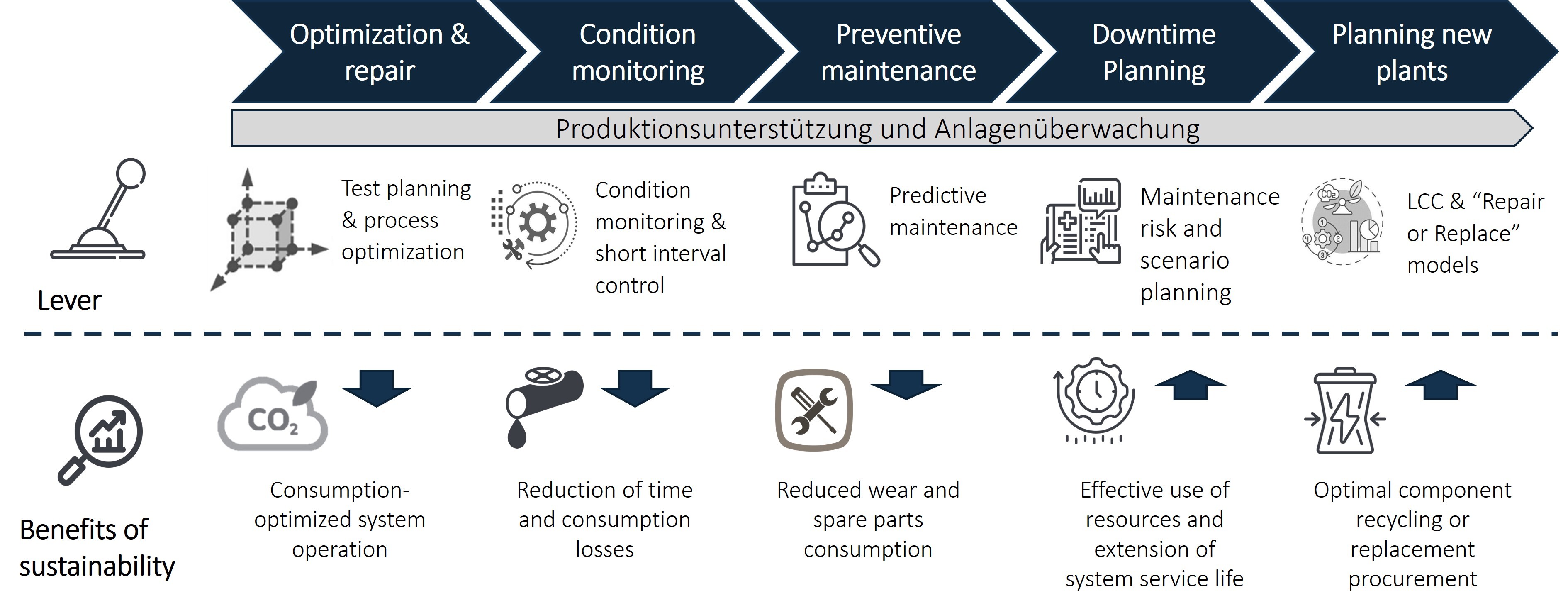
Figure 1: Key areas of action and levers for realizing hidden sustainability potential through the targeted use of AI in maintenance and asset management.
Predicting the future with AI: AI-supported algorithms for determining wear and tear and for inspection planning
A typical task of predictive maintenance is to generate useful forecasts from a large amount of data. One example is the creation of a reliable wear forecast for a critical system component. This is achieved through AI-supported modeling with the help of advanced analytics and the use of learning systems with dynamic algorithms to detect anomalies and problems. This extends the service life of critical components and interventions can be planned for the optimum time to avoid production downtimes.
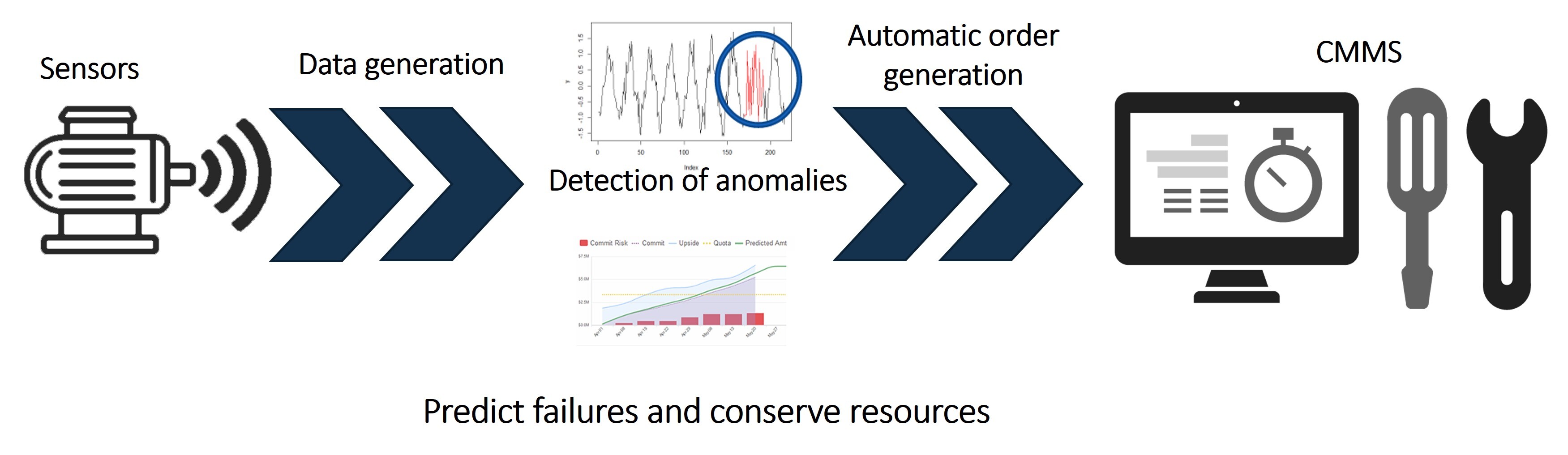
Figure 2: Key areas of action and levers for realizing hidden sustainability potential through the targeted use of AI in maintenance and asset management.
With better knowledge of the technical condition of critical systems, more targeted predictions can be made about potential system conditions and the associated risks and necessary revisions. With AI tools such as a Large Language Model, a real dialog with the system is possible. This makes it easier to analyze system statuses and initiate the right measures in good time.
On this improved information basis, various factors that are decisive for sustainability can be optimally combined, e.g.
- Frequency and intervals of downtimes
- Optimal duration and timing of downtime
- Sequences for shutdown and start-up of the subsystems
- Necessary system renewal or new systems
- Synergies or conflicts of the products to be produced
This planning approach makes it possible to make optimum use of resources, minimize downtime and at the same time considers the broader sustainability factors.
AI and digital twins: Optimization of system operation by determining the optimal, sustainable process parameters
Digital twins are a virtual representation of existing machines and components of a production system. Digital twins provide a real-time simulation of system operation and make it possible to test various operating modes and scenarios before they are implemented in production. The AI is so powerful that the behavior of systems, machines and individual components can be tested under different framework conditions and with different sustainability requirements.
AI-supported decision-making uses the data from the simulation to derive recommendations for action and determine the optimum process parameters. These parameters are used for optimum system control by specifying target values for different production requirements and system states.
A core topic of sustainable plant operation is the optimization of energy consumption: AI-controlled platforms for energy consumption analysis manage energy consumption company-wide. They forecast energy consumption and the necessary energy consumption and define time windows for optimum system operation in terms of sustainability, system availability and system efficiency. This enables them to achieve optimum productivity with minimized CO2-emissions.
AI-supported plant renewal increases the sustainability of the asset portfolio
A large number of factors must be taken into account when managing the plant portfolio and deciding whether ageing plants should be maintained or replaced: In addition to technical aspects, the central challenge is to manage the plant portfolio economically and sustainably.
AI-supported systems model plant operation and enable data-based decision-making that takes many parameters into account. A system condition assessment that also uses digital twins leads to the most economical and sustainable overall solution.
Digital twins simulate the operating life of systems based on historical sensor data and real wear models. AI-supported decision models use these simulations to calculate when a system can be economically maintained or should be replaced.
AI creates a new quality of transparency
The use of AI creates a new quality of transparency for industrial production through the quantity and quality of the information processed, increased analysis capability, the reliability of the results available and, finally, the speed with which companies can access these results anywhere and at any time.
This transparency enables companies to make better decisions, considering aspects such as profitability, sustainability and strategic perspectives for corporate development. ConMoto supports you in using the AI tools with which your company defines and achieves future goals. Talk to us!